By David R. Clough (@David_Clough1)
The 2021 Sveriges Riksbank Prize in Economic Sciences in Memory of Alfred Nobel was jointly awarded to David Card “for his empirical contributions to labour economics” and to Joshua Angrist and Guido Imbens “for their methodological contributions to the analysis of causal relationships.”
All three laureates pioneered approaches to making causal inferences from observational data. As we each learn in introductory research methods courses, causal inference from observational data is hard! We might observe a relationship between a variable X and an outcome Y, but it is hard to know whether X caused Y, whether Y caused X, or whether some alternate data-generating process produced the relationship.
In laboratory experiments, an experimenter can manipulate the antecedent variable X. By randomizing experiment participants into ‘treatment’ and ‘control’ groups, the experimenter can be confident that differences in outcomes were caused by their experimental intervention. In observational data, we can try an approximate an experiment if we find an environmental feature that causes variation in the explanatory variable X that is as good as random. This is what we mean by the phrase “natural experiment.” If we understand how the natural experiment caused variation in our explanatory variable, X, it helps us measure how our explanatory variable affects our outcome variables of interest.
While social scientists have long debated how to use natural experiments for causal inference, the contributions Angrist and Imbens made in the mid-1990s constituted a major step forward in how to think clearly about what natural experiments allow us to measure. They clearly defined the assumptions we need to make when using natural experiments for causal inference, and—with the concept of the Local Average Treatment Effect—they specified precisely how far we can generalize from one observational study. These are the contributions that the Nobel Prize committee sought to recognize.
Why does this prize matter to strategy scholars?
Strategy research, perhaps more so than nearly any other branch of social science, relies on archival, observational data for theory-building and theory-testing. With whole firms as the focal level of analysis, randomized, controlled experiments are often off-limits to strategy scholars. Many strategy researchers collect panel data on the whole population of firms in an industry. This type of data is particularly amenable to the use of natural experiments for causal identification, such as using region-specific policy changes to define treatment and control groups and measure difference-in-differences between the two groups. This approach has been employed to great effect by researchers studying strategy topics such strategic human capital resources and rates of firm-level innovation.
In strategy research we talk about developing theory about causal mechanisms. When we talk about “making a theoretical contribution” what we often mean is providing persuasive logical reasoning that helps us to understand (i) what causes what and (ii) why. In that sense, the contribution by Angrist and Imbens is at the heart of our theory-building and theory-testing process: they help to establish with precision what causal claims we can make from natural experiments. Angrist, and Imbens had neither the first word nor the last word when it comes to the longstanding debate over what causal inferences we can make from observational data. However, their work provided a massive leap forward in our thinking. While it took some time for the field of economics to absorb their findings, the techniques they helped refine became the bread-and-butter of applied economics in the early 2000s and have become a core part of the econometrics training many strategy scholars since that time have received.
To quantify the impact of the techniques Angrist and Imbens pioneered on strategy research, I used Google Scholar to collect some time-series data on how many articles in the Strategic Management Journal mention several phrases related to causal identification. As the figure shows, interest in causal identification generally—represented with the key phrases “instrumental variable,” “natural experiment,” and “field experiment”—has picked up gradually over the past 20 years. About 16% of SMJ articles from the last three years mention the phrase “instrumental variable.” However, the field’s adoption of the precise terminology of Angrist and Imbens —who carefully distinguish the “local average treatment effect” from other things we might want to estimate—appears somewhat slower.
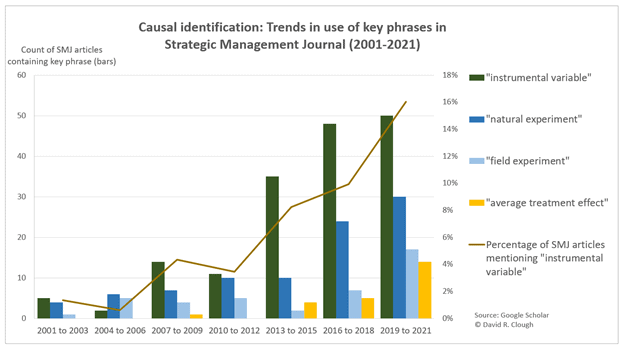
The approach pioneered by Angrist and Imbens has led to what is sometimes referred to as a ‘credibility revolution’ in social science. However, as with many innovations, the adoption of precise methods of causal inference has been gradual. Even when strategy scholars attend to problems of endogeneity and employ natural experiments and field experiments to assist with causal identification, it seems that we are not yet widely discussing exactly which treatment effect we are estimating (ITT versus ATE versus LATE). I therefore prefer to think of the field as undergoing a process of ‘methodological evolution.’ We are collectively learning over time to be careful what causal claims we make. We continue to value descriptive, non-causal research—both qualitative and quantitative—for the insights it can provide into rich and complex phenomena. And we are collectively shifting towards greater transparency in our methods so that others can replicate, rely on, and build on our findings in a cumulative manner.
If you have reflections on how research by Card or Angrist and Imbens has affected strategy research and you would like to contribute a post to this blog, please email me at david.clough@sauder.ubc.ca